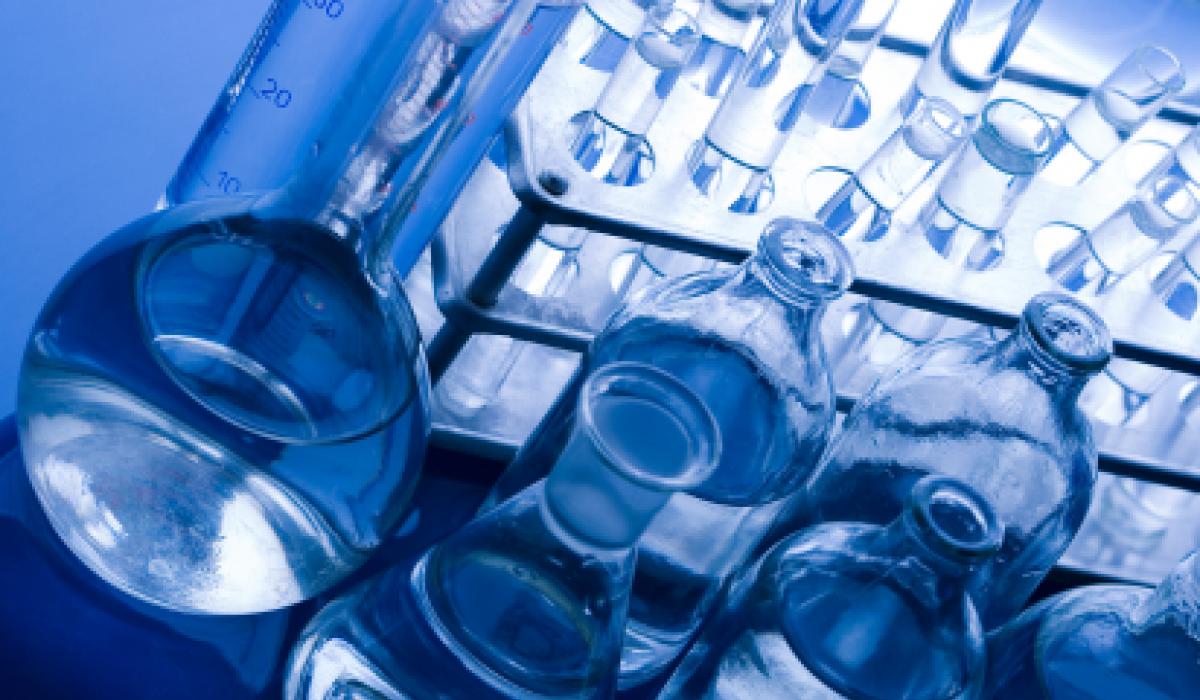
The University of Cincinnati Drug Discovery Center (UC DDC) has a physical library of 300,000 chemicals that have the general characteristics (i.e. size, chemical properties) of drug-like molecules, and knowledge regarding this library was made available to SAM. With the atomic coordinates of the target protein available, the next step was to predict the inhibitory potential of each of 1,200,000 chemical states derived from the library against PfMAP2. In principle, this can be done using a variety of methods; the most prominent ones for an application of this scale are molecular docking, ligand-based virtual screening and pharmacophore- based virtual screening. In molecular docking the binding of a chemical to a target protein is simulated, and the binding free energy, and thus the inhibitory potential, is estimated using a ‘scoring function’.
The underlying principle of ligand-based virtual screening is that similar chemicals tend to have similar biological effects. If knowledge about an inhibitor of the target protein exists one can calculate the chemical similarity of each molecule in a screening library, and use this information to predict potential new inhibitors. Pharmacophore-based screening is related to ligand-based screening, but it includes the additional information that not every part of a chemical is equally important for the biological activity of that chemical. A pharmacophore is the abstract definition of the pattern of molecular features that are necessary for the biological activity of a chemical against one particular protein target. A chemical library can then be searched for members that match these molecular features, which indicates the potential for similar activity. Because there was no known inhibitor of PfMAP2 we could not initially use ligand-based virtual screening methods. Also, for pharmacophore-based screening, knowledge of existing active molecules is beneficial, although not strictly necessary.
Therefore, it was decided to base our computational predictions solely on molecular docking calculations in the first instance. To increase the reliability of the docking predictions we decided to use three different, independent docking methods and to combine their results to reach a consensus prediction. So, three docking-based virtual screens were carried out, one using the software AutoDock and two using the software packages Vina and Glide. From the combined results we selected 1031 predicted ‘hits’. The chemical identifiers of these hits were passed on to test these chemicals experimentally for their inhibitory power against PfMAP2. Meanwhile, we created a pharmacophore-based model based on existing background knowledge of kinase–ligand interactions combined with a consideration regarding unique features of PfMAP2. The method identified 588 chemicals from the library that fitted the pharmacophore features and were therefore predicted as ‘hits’. The chemical identifiers of these molecules were also added to the testing library for subsequent laboratory work.