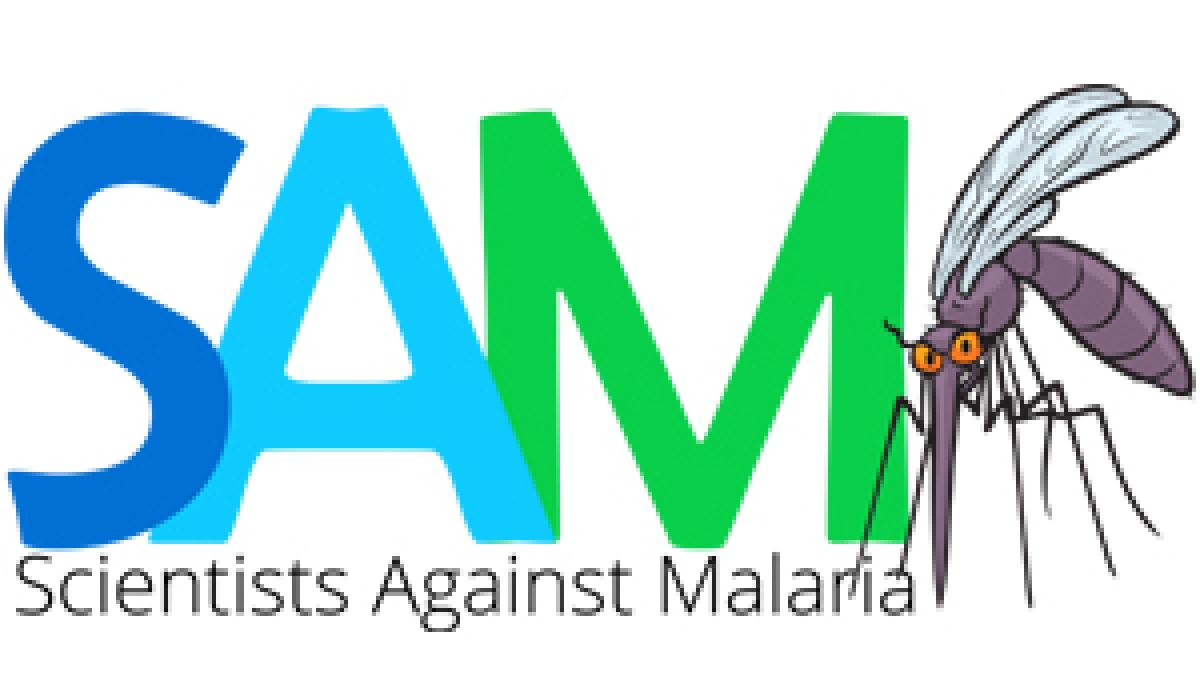
The Scientists Against Malaria (SAM) consortium was formed in 2010 by Douglas Connect (DC) from the InnovationWell Neglected Diseases Collaboration Pool as a virtual drug discovery organization to collaborate on the design of kinase inhibitors against the P. falciparum malarial parasite. In theory, to find a drug that is active against a chosen protein target it would be sufficient to test every possible candidate chemical to see if it substantially inhibited the target protein’s function. Hit molecules would then be tested for potential toxic or adverse effects in humans, to ensure that they are only active against the target protein, but not against human proteins.
In practice, however, there are several obstacles to this simple endeavor. First, the number of possible candidate chemicals is prohibitively large to be able to experimentally test every one (let alone obtaining or synthesizing them all), especially within a Virtual Organization (VO) such as SAM, working with a limited budget. Compound libraries used by pharmaceutical companies for drug discovery often contain a few to several million molecules. The second aspect ensuring the absence of human toxicity is even more difficult to test. Usually, animals are used as substitutes for humans, and from the effects that the drug candidates have on these animals attempts are made to estimate the effects they would have on humans. However, this process is costly and prone to complications. To reduce the time and costs needed to carry out experimental tests on a large scale, computational predictions are increasingly used in many drug discovery applications, both for predicting the activity of chemicals against the chosen protein target, and for predicting potential the human toxicities of candidate molecules.
The computational prediction of these biological activities is, however, very difficult and often not very accurate, especially when addressing new problems where knowledge is limited. Many good methods exist, but they take significant resources and long computing times, which limits their current application to large compound libraries. Methods that are rapid enough for such large-scale applications often are rather inaccurate; therefore, computational methods are usually not applied to replace experimental methods, but rather act in a complementary way for prioritization, limiting the vast number of chemicals inside screening libraries to a size that is more easily managed experimentally.